pipeline_tag: text-generation
|bosbos-2-7b|
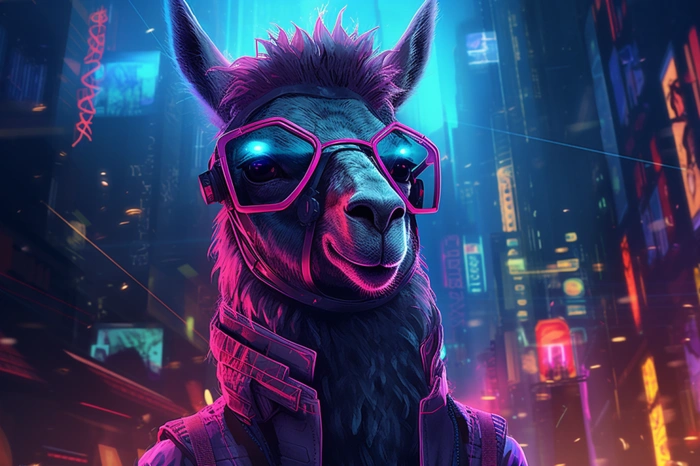
This is a llama-2-7b-chat-hf
model fine-tuned using QLoRA (4-bit precision) on the mlabonne/guanaco-llama2
dataset.
🔧 Training
It was trained on a Google Colab notebook with a T4 GPU and high RAM.
💻 Usage
# pip install transformers accelerate
from transformers import AutoTokenizer
import transformers
import torch
model = "bosbos/bosbos-2-7b"
prompt = "what is prediction in frensh ?"
tokenizer = AutoTokenizer.from_pretrained(model)
pipeline = transformers.pipeline(
"text-generation",
model=model,
torch_dtype=torch.float16,
device_map="auto",
)
sequences = pipeline(
f'<s>[INST] {prompt} [/INST]',
do_sample=True,
top_k=10,
num_return_sequences=1,
eos_token_id=tokenizer.eos_token_id,
max_length=200,
)
for seq in sequences:
print(f"Result: {seq['generated_text']}")
Or use this :
# !pip install -q accelerate==0.21.0 peft==0.4.0 bitsandbytes==0.40.2 transformers==4.31.0 trl==0.4.7
import torch
from transformers import (
AutoModelForCausalLM,
AutoTokenizer,
BitsAndBytesConfig,
pipeline,
)
###############################################################################
# bitsandbytes parameters
################################################################################
# Activate 4-bit precision base model loading
use_4bit = True
# Compute dtype for 4-bit base models
bnb_4bit_compute_dtype = "float16"
# Quantization type (fp4 or nf4)
bnb_4bit_quant_type = "nf4"
# Activate nested quantization for 4-bit base models (double quantization)
use_nested_quant = False
################################################################################
# SFT parameters
################################################################################
# Maximum sequence length to use
max_seq_length = None
# Pack multiple short examples in the same input sequence to increase efficiency
packing = False
# Load the entire model on the GPU 0
device_map = {"": 0}
model_name="bosbos/bosbos-2-7b"
# Load tokenizer and model with QLoRA configuration
compute_dtype = getattr(torch, bnb_4bit_compute_dtype)
bnb_config = BitsAndBytesConfig(
load_in_4bit=use_4bit,
bnb_4bit_quant_type=bnb_4bit_quant_type,
bnb_4bit_compute_dtype=compute_dtype,
bnb_4bit_use_double_quant=use_nested_quant,
)
# Load base model
model = AutoModelForCausalLM.from_pretrained(
model_name,
quantization_config=bnb_config,
device_map=device_map
)
model.config.use_cache = False
model.config.pretraining_tp = 1
# Load LLaMA tokenizer
tokenizer = AutoTokenizer.from_pretrained(model_name, trust_remote_code=True)
tokenizer.pad_token = tokenizer.eos_token
tokenizer.padding_side = "right" # Fix weird overflow issue with fp16 training
# Run text generation pipeline with our next model
prompt = "what is prediction in frensh ?"
pipe = pipeline(task="text-generation", model=model, tokenizer=tokenizer, max_length=200)
result = pipe(f"<s>[INST] {prompt} [/INST]")
print(result[0]['generated_text'])
Output:
"Prédiction" is a noun that refers to the act of making a forecast or an estimate of something that will happen in the future. It can also refer to the result of such a forecast or estimate.
For example:
- "La prédiction de la météo est que il va pleuvoir demain." (The weather forecast is that it will rain tomorrow.)
- "La prédiction de la course de chevaux est que le favori va gagner." (The prediction of the horse race is that the favorite will win.) In English, the word "prediction" is often used in a similar way, but it can also refer to a statement or a prophecy about something that has already happened or is happening.
- Downloads last month
- 5
This model does not have enough activity to be deployed to Inference API (serverless) yet. Increase its social
visibility and check back later, or deploy to Inference Endpoints (dedicated)
instead.